Autonomous driving has attracted tremendous attention, especially in the past few years. Many technologies are required but robust 3D perception is an essential prerequisite for interacting with the environment. Currently, the most standard approaches are based on LIDAR for detecting and recognizing objects, for discovering drivable road, and related tasks. But 3D perception from visual information, such as images or videos, is also important for reducing cost. Moreover, it raises interesting challenges which are of particular interest to the computer vision community. The prerequisite for building a successful 3D vision system is a large amount of training and testing data with annotations of the 3D ground truth, including depth, 3D object shapes, and semantic properties. But these quantities are typically very difficult to annotate, which hinders the development of computer vision algorithms to overcome these challenges.
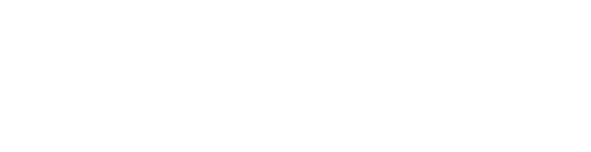